Descriptive and Inferential Statistics
- Post author:Alex Uriarte
- Post published:May 23, 2021
- Post category:Fan
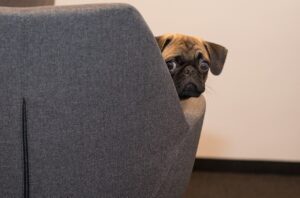
Since I posted “Challenges in Exploratory Data Analysis” (February 1, 2021), I found myself struggling with the distinction between Exploratory Data Analysis and Confirmatory Data Analysis on one hand, and the distinction between Descriptive Statistics and Inferential Statistics on the other. The former distinction is relevant to what you can say with any one set of data and what you can say with more than one data set; while the latter distinction comes into play when deciding whether our interest lies in the sample at hand or on the process generating the sample we have (the population).
Clarifying these distinctions is more than an academic exercise: doing so, and understanding how the terms are used, help us understand what we can say with the data and what we cannot, what assumptions we are making when inferring from the data and at what point in our analysis we are making those assumptions. It helps develop our own guidelines for disciplining our thought process when thinking with data.
According to Wikipedia (Wikipedia contributors 2021a), Exploratory Data Analysis was promoted by US mathematician John Tukey in the 60s and 70s, as a way of unearthing hypotheses to be tested with data before jumping onto testing hypotheses based on assumptions made. It was to be in contract with Confirmatory Data Analysis (hypothesis testing). It was a way of exploring what information was contained in the data, independent of any already existing hypotheses about the relevant subject matter. It included a myriad of techniques such as looking at variable maximums, minimums, means, medians and quartiles, but was characterized more by the attitude than the techniques. A number of techniques applied in exploratory data analysis can be applied whether our focus is on the sample at hand (descriptive statistics) or the underlying generating process (inferential statistics). In thinking about these concepts, I produced the diagram below, that is useful to me, may be useful to others as well (I used mostly my accumulated knowledge at this point, but suggest readers start with Wikipedia entries for Descriptive Statistics [2021b] and Statistical Inference [2021c] for further reading).
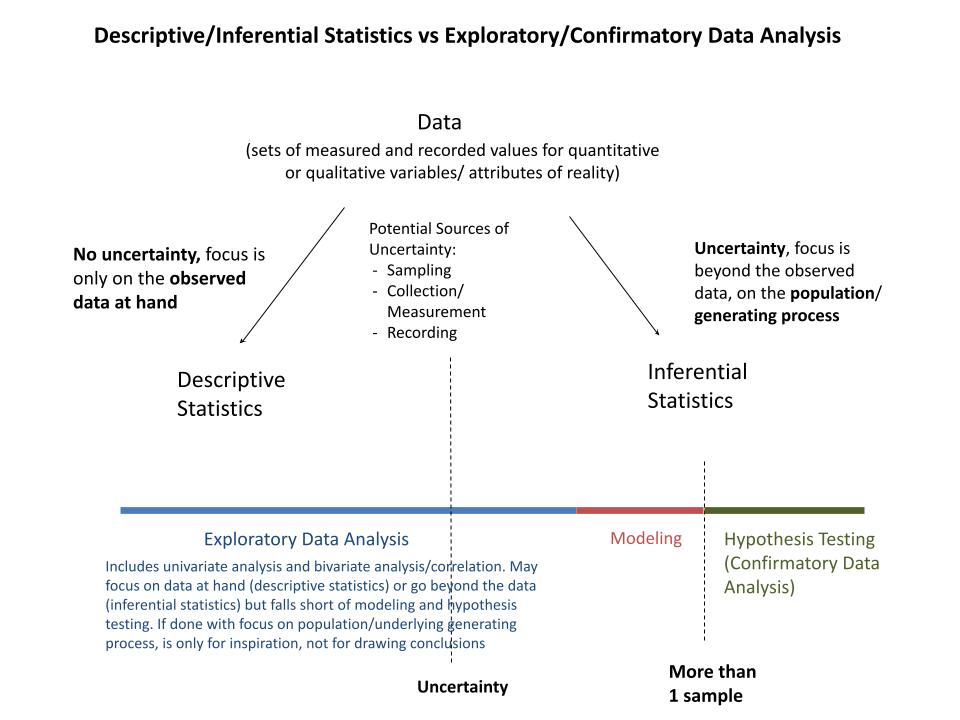
Although exploratory data analysis techniques can be applied whether our focus is on the sample at hand or the underlying generating process, how things are done in each case may be different. In the table below I tried to establish some distinctions on how we would proceed with exploratory data analysis in descriptive and inferential statistics.
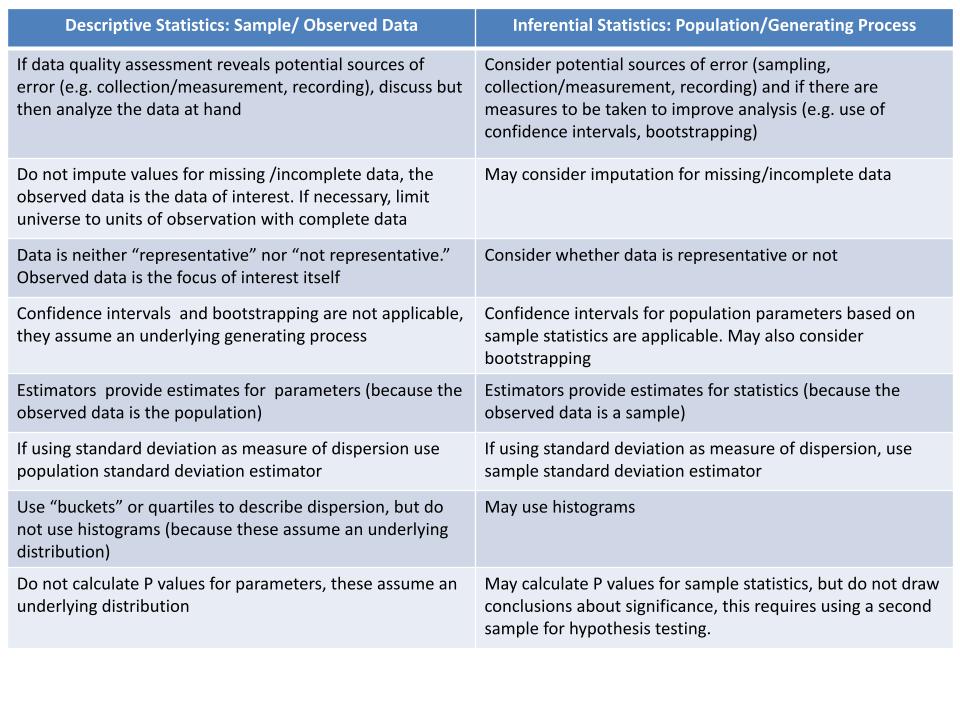
In either case, during exploratory data analysis, we do not talk about significance of correlation, causality or hypothesis testing. These require modeling and a second sample drawn from the same population (or treatment and control groups).
A final note on the terms used by Cassie Kozyrkov in her popular blogs and vlogs (Kozyrkov 2018; 2019a; 2019b; 2020). She refers to data analytics as being used when there is no uncertainty (what I refer to as descriptive statistics) and refers generally to statistics when there is uncertainty (what I refer to inferential statistics). She also refers to data analytics as being for inspiration (what I refer here as exploratory data analysis), as opposed to hypothesis testing, that would require another sample. From what I can tell from the literature, these are less traditional uses of the terms and I find the traditional uses (what I believe I capture here) seem to better highlight the difference between analyzing sample and population data.
References
Kozyrkov, Cassie. 2018. “Don’t Waste Your Time on Statistics.” Towards Data Science. May 29. Available: https://towardsdatascience.com/whats-the-point-of-statistics-8163635da56c. Accessed: May 23, 2021.
———-. 2019a. “Statistics for People in a Hurry.” Towards Data Science, May 29. Available: https://towardsdatascience.com/statistics-for-people-in-a-hurry-a9613c0ed0b. Accessed. May 23, 2021.
———-. 2019b. “The Most Powerful Idea in Data Science.” Towards Data Science. August 09. Available: https://towardsdatascience.com/the-most-powerful-idea-in-data-science-78b9cd451e72. Accessed: May 23, 2021
———- 2020. “How to Spot a Data Charlatan.” Towards Data Science. October 09. Available: https://towardsdatascience.com/how-to-spot-a-data-charlatan-85785c991433. Accessed: May 23, 2020.
Wikipedia contributors, 2021a.”Exploratory data analysis.” In Wikipedia, The Free Encyclopedia. Available: https://en.wikipedia.org/w/index.php?title=Exploratory_data_analysis&oldid=1021890236. Accessed May 15, 2021.
———-. 2021b. “Descriptive Statistics.” In Wikipedia; The Free Encyclopedia. Available: https://en.wikipedia.org/wiki/Descriptive_statistics. Accessed May 23, 2021.
———-. 2021c. “Statistical Inference.” In Wikipedia; The Free Encyclopedia. Available: https://en.wikipedia.org/wiki/Statistical_inference. Accessed May 23, 2021.