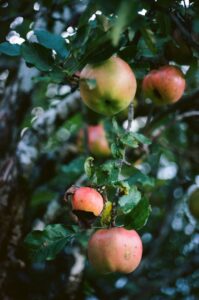
On a recent trip to the local public library I happened to find a copy of a book of collected works by Bertrand Russel that I used to own but that, as many other books, had been a victim of my international moves. I always admired Bertrand Russel’s clear, simple and straightforward way of discussing not so simple topics without distorting them (at least not in ways that were obvious to me). The library was selling the book as part of a used book sale and I bought it, together with a copy of Bertrand Russel’s “The Scientific Outlook.”
In reading this latter book, I found myself sucked into a web of interrelated methodological discussions; some old ones (e.g. how scientific the social sciences are or can be) and some newer ones (e.g. whether the huge amount and speed of data availability, and easy access to it, brought by information technology, has challenged traditional scientific methodology and put correlation – with no theory – front and center on the research agenda). I remember delving into economic methodological discussions some thirty years ago as an economics student but have distanced myself from economic theory since. After getting lost in the rabbit hole, I decided I did not have enough time to dig deep enough into these discussions, but thought I would register what I found, perhaps for continuing/revisiting at a later date.
So here goes.
Both Mlodinow (2009) and Russel (1962) place the origins of the scientific revolution in the late sixteenth and early seventeenth century, pretty much on the shoulders of Galileo Galilei (1564-1642), his contemporaries and those coming soon after him (e.g. Isaac Newton – 1643-1727). They also both characterize the scientific revolution as being centered on induction and experimentation, as opposed to deduction, as a source of knowledge. Both deduction (theory) and induction (evidence) have a role in science and Russel describes the scientific method as including three stages:
Observing significant facts
Arriving at a hypothesis, which, if true, would account for these facts
Deducing from this hypothesis consequences which can be tested by observation (some, quoting Karl Popper, would say “refuted” by evidence)
This characterization of the scientific method (and its variations) seems to have been criticized over time as not adequately portraying how science evolves. The idea that science progresses by refuting hypotheses empirically, for example, seems to have been criticized repeatedly over time. A recent opinion article in Scientific American (Singham 2020) claims that it must be abandoned for good for at least two reasons: first, because empirical experiments are framed by many theories themselves making its results more reflective of comparisons between theories than between theory and evidence; second, because this is not really how science has advanced historically. Rather, the author claims, “It is the single-minded focus on finding what works that gives science its strength, not any philosophy.“ Similar arguments have been made by various philosophers of science, including Thomas Kuhn (Wikipedia 2021).
The use of empirical evidence may vary from one branch of science or research program to another. I particularly looked for discussions among economists, because that is an area I have more of a background in and because of its relevance to international development. In a well known paper, Larry Summers (1991) argues that elaborated statistical tests aimed at estimating model parameters have had little consequence to advance economic thinking, that most papers remembered as having advanced economic theory have little empirical content at all, and that successful empirical research in economics have relied mostly on attempts to gauge strength of association and on persuasiveness. He criticizes models that have been overspecified to enable testing under the argument that results tend be of little worth and, comparing economics to natural sciences he states that “The image of an economic theorist nervously awaiting the result of a decisive econometric test does not ring true.”
In general, the Popperian criteria of falsifiability through testing seems to be simultaneously nominally accepted and yet in practice not met in economics, with theory moving forward anyway, based on the use of empirical evidence to support argumentation. Hausman (2018) summarizes the challenges of application of Popperian criteria to economics (presumably applicable to the social sciences more generally) and how several authors have abandoned completely the criteria to argue that economics (and, again, presumably the social sciences more generally) advances by using a more comprehensive blend of theory and empirical evidence. Durlauf (2012) states that “while some empirical economics involves the full delineation of an economic environment, so that empirical analysis is conducted through the prism of a fully specified general equilibrium model, other forms of empirical work use economic theory in order to guide, as opposed to determine, statistical model specification. Further, a distinct body of empirical economics is explicitly atheoretical, employing so-called natural experiments to evaluate economic propositions and to measure objects such as incentives.
A more recent discussion on the use of evidence to advance our knowledge of society gained traction with the rapid growth of “big data. ” Some claimed that data in large volume would make the scientific method obsolete and the correlation would suffice to advance our knowledge, even if these claims may come from outside the academic community. For example, an article in WIRED magazine, written by its Editor in Chief, claimed that “Petabytes allow us to say: ‘correlation is enough.’ We can stop looking for models. We can analyze the data without hypotheses about what it might show. We can throw the numbers into the biggest computing clusters the world has ever seen and let statistical algorithms find patterns where science cannot” (Anderson 2000). These kinds of claims have been countered by others (e.g. Mazzochi 2015) and it is hard for me to imagine how computerized analysis of data would not be imbued with human theorizing, no matter how much one tries to step aside and let “data speak.” In addition, for my purposes, much of the data in international development is not “big data.” Even if it were, it is not clear to me how we would separate the many variables that in international development tend to move together (in the same or opposite direction) with just correlation as a criteria (and no theory).
There is a large literature to review on this topic and I have not even looked at the random control trial based research that gave Esther Duflo, Abhijit Banerjee and Michael Kremer the 2019 Nobel prize in economics, and what that line of research means for the discussion above. But I am thinking (for now) that there may not be a clear rule in the use of evidence and theory for discussing international development knowledge, and I am satisfied (for now) with looking for the reasonable use of theory, evidence, skepticism and caution in thinking of development policy and practice. I am sure I will come back to this discussion at a later date.
References:
Anderson, Chris. 2000. The End of Theory: The Data Deluge Makes the Scientific Method Obsolete. WIRED. June. Available: https://www.wired.com/2008/06/pb-theory/. Accessed: October 30, 2021.
Boland, Lawrence. 2006. Seven decades of economic methodology: a Popperian perspective. In: Karl Popper: a Centenary Assessment: Science, I. Jarvie, K. Milford and D. Miller (Eds), 2006, 219–27. Available: http://www.sfu.ca/~boland/wien02.pdf. Accessed: October 30, 2021
Durlauf, Steven. 2012. Complexity, Economics, and Public Policy. Politics, Philosophy & Economics 11(1) 45–75. Sage. Available: http://home.uchicago.edu/sdurlauf/includes/pdf/Durlauf%20-%20Complexity%20Economics%20and%20Public%20Policy.pdf. Accessed: October 30, 2021.
Hausman, Daniel. 2018. Philosophy of Economics. Stanford Encyclopedia of Philosophy. Available: https://plato.stanford.edu/entries/economics/#RhetEcon. Accessed: October 30, 2021
Mazzocchi, Fulvio. 2015. Could Big Data be the end of theory in science? A few remarks on the epistemology of data-driven science. EMBO reports. EMBO Press. Available: https://www.ncbi.nlm.nih.gov/pmc/articles/PMC4766450/pdf/EMBR-16-1250.pdf. Accessed: October 30, 2021.
Mlodinow, Leonard. 2009. The Drunkard’s Walk. How Randomness Rules Our Lives. New York: Vintage Books. A Division of Random House.
Russel, Bertrand. 1962 (first copyrighted in 1931). The Scientific Outlook. The Norton Library. W.W. Norton & Company.
Singham, Manu. 2020. The Idea That a Scientific Theory Can Be ‘Falsified’ Is a Myth. It’s time we abandoned the notion. In Scientific American, September 2020. Available: https://www.scientificamerican.com/article/the-idea-that-a-scientific-theory-can-be-falsified-is-a-myth/. Accessed: October 30, 2021
Summers, Larry. 1991. The Scientific Illusion in Empirical Macroeconomics. The Scandinavian Journal of Economics. Vol. 93, No. 2, Proceedings of a Conference on New Approaches to Empirical Macroeconomics (Jun., 1991), pp. 129-148 (20 pages). Wiley. Available: http://faculty.econ.ucdavis.edu/faculty/kdsalyer/LECTURES/Ecn200e/summers_illusion.pdf. Accessed: October 30, 2021.
Wikipedia contributors. 2021. Scientific Method. Available: https://en.wikipedia.org/wiki/Scientific_method. Accessed: October 30, 2021