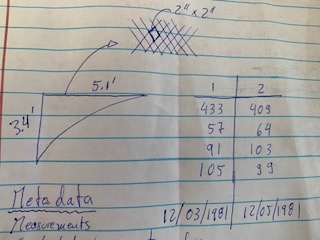
A few weeks ago I watched a few of Crash Course’s Data Literacy elearning videos on YouTube (Arizona State University and Crash Course 2020). It’s first episode defines “data” as “specific information we collect to make decisions.” This is a different definition from others I have heard. It does have some interesting aspects to it. Under this definition:
- Data would be a subset of information. That is, all data would be information but not all information data.
- It uses collection and decision making to define what information is data and what is not.
Other definitions are very different.
A common distinction between data and information is that found in the so-called DIKW pyramid or similar representations. DIKW stands for Data – Information – Knowledge – Wisdom, and usually suggests a hierarchy where data is the broader concept that is then filtered as information, that is in turn filtered as knowledge and finally as wisdom. This seems to be commonly used in the knowledge management community and is often attributed to an article by Russell Lincoln Ackoff in the Journal of Applied Systems Analysis in 1989 (e.g., see Bernstein 2009)
Under this representation, data are often interpreted as facts, noise or signals. There are many criticisms to this representation, from whether “filtering” is actually a good way of thinking about the connections between these concepts, to proposed changes to the pyramid, to what is actually the broader concept, data or information (for just a few examples of a relatively large literature, see Weinberger 2010; Tuommi 1999; and Dammann 2018).
Yet a third way of thinking about data is the definition contained in US law. US Federal statutes define data as “recorded information, regardless of form or the media on which the data is recorded” (44 U.S. Code § 3502). The definition is less innocuous than what it may seem at first. Recording information is in good part what distinguishes our handling of information from cultures who rely (or relied) on voice of mouth transmission and the potential loss of content associated with such practices: think of the telephone game that kids play, whispering a sentence in another one’s ear, who then whispers to another one, and so on until the last child states out loud what his/her understanding of the sentence is, often to find the sentence arrived at the end of the communication chain completely altered or distorted. Under this definition, however, information is the broader concept.
The table below summarizes the three different definitions of “data.”
ASU and Crash Course 2020 | U.S. Federal Statutes | DIKW pyramid | |
Definition or understanding | Specific information we collect to make decisions | Recorded information, regardless of form or the media on which the data is recorded | Facts, noise, signals |
Highlight | Data has a purpose: decision-making | Data must be recorded | Data as facts, no specific purpose or characteristic |
Data relative to information | Information > Data | Information > Data | Data > Information |
I do not find the last row – showing the relation between data and information – particularly useful in understanding data: it is a result of how we define not just data but also information, and may be more useful for discussions focused on knowledge. I include it in the table only for the sake of comparison and may explore it in other posts. I do find the “highlight” of each definition useful in thinking about data, how to manage and use them:
- Data should reflect facts. Whether it does or not, depends on how it was collected and managed. This is important to keep in mind in discussions about data collection, data curation and trusted repositories.
- Data should be recorded. This reinforces the importance of data curation and particularly of metadata in enabling us to understand what “facts” do the data actual capture.
- Data may be used for decision making. Hence, it is important to keep this in mind the many considerations around data bias, completeness, presentation and interpretation.
In this blog, I will use the highlight of each of the three definitions to discuss data.
References
44 U.S. Code § 3502. Legal Information Institute. Cornell Law School. Available: https://www.law.cornell.edu/uscode/text/44/3502#:~:text=(A)%20means%20the%20obtaining%2C,or%20format%2C%20calling%20for%20either%E2%80%94. Accessed: November 14, 2020
Arizona State University and Crash Course, 2020. Study Hall: Data Literacy. Available: https://www.youtube.com/watch?v=0H8awA3GBPg&list=PLNrrxHpJhC8m_ifiOWl1hquDmdgvcviOt&index=14. Accessed: November 27, 2020
Bernstein, J. H., 2009. The Data-Information-Knowledge-Wisdom Hierarchy and its Antithesis. In: Proceedings from North American Symposium on Knowledge Organization. Vol. 2. Available: https://journals.lib.washington.edu/index.php/nasko/article/viewFile/12806/11288. Accessed: November 27, 2020.
Dammann, Olaf. 2018. Data, Information, Evidence, and Knowledge: A Proposal for Health Informatics and Data Science. In: Online Journal of Public Health Informatics10(3):e224. Available: https://www.ncbi.nlm.nih.gov/pmc/articles/PMC6435353/pdf/ojphi-10-e224.pdf. Accessed: November 27, 2020.
Tuommi, Ikka. 1999. Data is more than knowledge: Implications of the reversed knowledge hierarchy for knowledge management and organizational memory. In: Journal of Management Information Systems 16(3):103-117.Available: https://www.researchgate.net/publication/328803142_Data_is_more_than_knowledge_Implications_of_the_reversed_knowledge_hierarchy_for_knowledge_management_and_organizational_memory. Accessed: November 27, 2020
Weinberger, 2010. The Problem with the Data-Information-Knowledge-Wisdom Hierarchy. In: Harvard Business Review. Available: https://hbr.org/2010/02/data-is-to-info-as-info-is-not. Accessed: November 27, 2020.