A Thought After Reading Daniel Kahneman’s “Thinking, Fast and Slow”
- Post author:Alex Uriarte
- Post published:February 13, 2021
- Post category:Fan
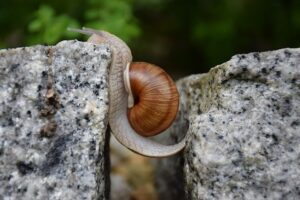
I can tell that Daniel Kahneman’s book, Thinking, Fast and Slow, is one of those books that I will find myself coming back to over and over again. Why? On one hand, it provides evidence from experimental psychology for phenomena that I was already inclined to believe, that is, it appeals to my own confirmation bias. At the same time, it offers a number of insights and explanations that are new to me and eye opening. Kahneman, a psychologist, won a Nobel Prize in Economics for his work on decision-making under uncertainty. His book reflects a lifetime of learning about human judgement and is an absolute delight to read.
It’s central framework seems to revolve around the idea that our minds address problems in two distinct ways, which he describes using the metaphors of System 1 and System 2. The table below summarizes some of the characteristics of each system.
System 1 | System 2 |
Fast | Slow |
Intuitive | Deliberate |
Automatic, cannot be turned off at will | Effortful and/but/therefore lazy |
Impulsive | Requires self-control |
Associative and causal, but does not have the capacity for statistical thinking | Requires training for statistical thinking |
Prone to bias and to believing | In charge of doubting and unbelieving |
Much of Kahneman’s book is then focused on System 1 and its characteristics and biases. We learn of its need for coherence and the central role of cognitive ease in driving belief. System 2 is much less explored in the book and I was left with the desire to learn more about what can be done to strengthen our use of System 2.
Why would we want to strengthen our reliance on System 2? It is not obvious that doing so would necessarily lead to better social outcomes, if we are willing to rely on expertise.System 1’s intuitive thought draws both on heuristics (shortcuts that replace a more complex problem with a simpler one) and on expertise (recognition of information previously acquired). Becoming an expert means you can draw on your acquired information to reach better conclusions with less effort. In other words, the more we rely on experts, the more likely we are to avoid incorrect conclusions in a world governed by System 1 thinkers.
However, we are unlikely (and it is probably impossible) to seek expertise in the myriad of problems and decisions we encounter on a daily basis. And as Kahneman points out in a reference to the work of another psychologist, Daniel Gilbert, System 1 is prone to believe (Kahneman, 2011, Chapter 7). “Unbelieving” is the effortful task of System 2. If we want to form opinions and draw conclusions about much of the information we encounter on any given day, we will need to spend energy, potentially a lot of energy: we will need to be willing to lead an effortful, even if potentially fulfilling life. Those less susceptible to the System 1 biases and more prone to calling on System 2 Kahneman calls “engaged.”
Should we strive to be more “engaged?” If so, how?
References
Kahneman, Daniel, 2011. Thinking, Fast and Slow. Farrar, Straus and Giroux.