Similarities in GDP Per Capita Trajectories
- Post author:Alex Uriarte
- Post published:March 5, 2023
- Post category:Engelberg Huller
[For the data and R code for this blog post, please visit my GitHub repository EngelbergHuller/GDP-Growth-Similarities]
In my previous Engelberg Huller post, “Catch-up,” January 20, GDP per capita growth data over a 60 year period seems to suggest similarities in growth trajectories of countries geographically close to each other, whether reflecting similar institutions and histories, economic integration and interdependency patterns, or some other factor.
In an attempt to further explore these similarities, but also teach myself a bit of the open source statistical software R, I decided to look at growth data using an R package called “Similarity Measures.” This package offers functions built to compare two vectors and assess the numerical proximity between the elements of those vectors. Functions such as these are often used to compare the distance between two geographical trajectories, such as those of migrating animals or traffic. But they can also be used to compare trajectories of single variables over time.
I used the same dataset of Gross Domestic Product in constant local currency units over the 60 year 1961-2020 period that I used in the “Catch-up” post. I had to exclude 3 of the 93 countries used in “Catch-up” for lack of complete data for all the 60 years and I transformed GDP in constant LCUs into an index with 1961 = 100 to be able to compare trajectories in the same unit of measurement.
I used a function called Longest Common Subsequence (LCSS). This function counts the number of elements that are considered equivalent under certain criteria. The criteria are determined by three parameters. The following is my understanding of these parameters:
- The first establishes what elements in each vector are compared. In the R function, this is the “pointSpacing” argument, A value of 2 means that 2 intervals between the indexed elements of each vector are allowed.
- The second parameter establishes the difference allowed in the values between elements compared, for those elements to be considered equivalent. In the R function, this is the “pointDistance” argument.
- The third parameter I have less of an understanding but is a margin of error established for the algorithm calculations, and it influences the “accuracy and speed of the calculation.” In the R function, this is the “errorMarg” argument. In calibration, this parameter seemed to make little difference in the outcomes.
I initially applied the LCSS function to the country GDP per capita index trajectories where 1961 was set to 100 for all countries. Because the LCSS function compares years based on the distance between their values, there are many more years considered the same by the function in the early period of the trajectories (say, 1960s) than in the later period (say, 2010s). This is not what we would like. We would like all period of the trajectories to be valued the same when accessing similarity between two trajectories.
So I turned to applying the LCSS function on the growth rates themselves. Doing so would mean that, when one countries GDP per capita index trajectory goes up, say 3 percentage points, and another one does too, the two trajectories would be considered equivalent in that year, even if at that point in time their cumulative growth histories had distanced their growth trajectories.
To calibrate the LCSS function (choose the parameters to use), I used the trajectories for Argentina and Uruguay, two countries whose GDP per capita growth trajectories appeared to be closely related in my “Catch-up” post. I chose parameters that seemed intuitively reasonable and that didn’t seem to generate extreme outcomes (e.g. entire trajectories for two countries being considered the same or only the first year, 1961 = 100, being considered the same). I ended up with:
- pointSpacing = 2
- pointDistance = 2
- errorMarg = 0.5
Running the LCSS function to compare 90 countries, 2 by 2, in all possible combinations, generates a matrix with 8100 results with diagonal = 59 (each country’s trajectory when compared to itself shows all 59 years being equivalent). This leaves 8100-90 = 8010 results that compare different countries. Because the function compares, say, Argentina to Uruguay and then Uruguay to Argentina, the number of unique results comparing two different countries is actually 8010/2 = 4005. Because it took my laptop a few seconds to compare each pair of trajectories, running the function for the entire set of 90 countries took me over 11 hours (and so I did each run overnight).
Out of the 90 countries, the two that had the closest GDP growth trajectories were France and Austria. With the parameters chosen, their growth rates were equivalent in 58 of the 59 years. The least similar trajectories had growth rates that were equivalent in 20 of the 59 years and there were three pairs of trajectories with that score: Burma and Bahamas, Greece and Chad, Iran and Indonesia.
The eight most similar pairs of trajectories were among five European countries: France, Belgium, Netherlands, Italy and Austria. Their growth trajectories are shown in Figure 1, below.
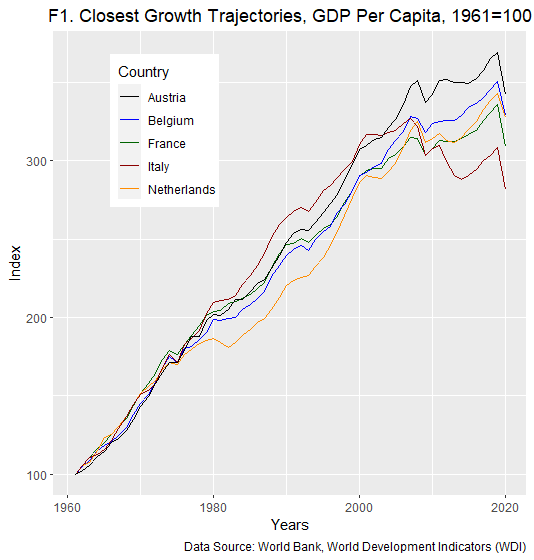
Figures 2 shows the most similar GDP per capita trajectories, those of France and Belgium, and Figure 3 shows their growth rates.
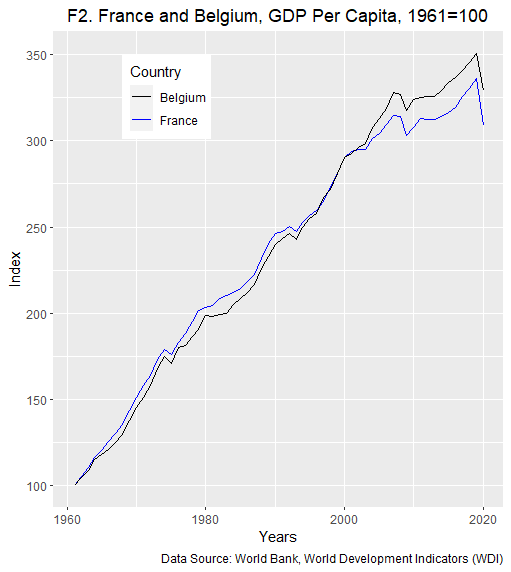
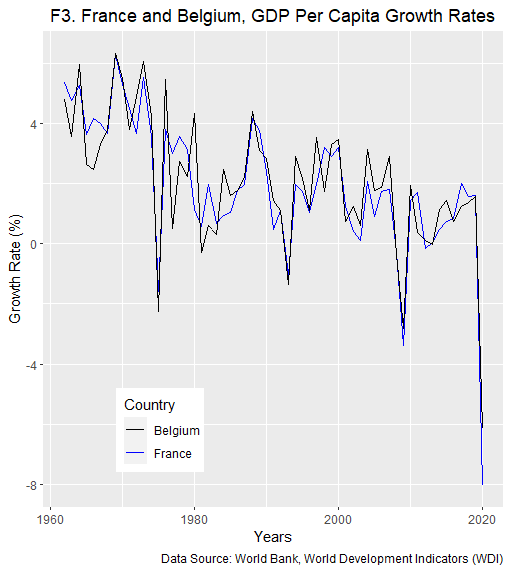
The South America Southern Cone had GDP per capita similarity scores in the 20s and 30s, i.e. their growth rates were similar in 20 to 40 of the 59 years compared (F4)
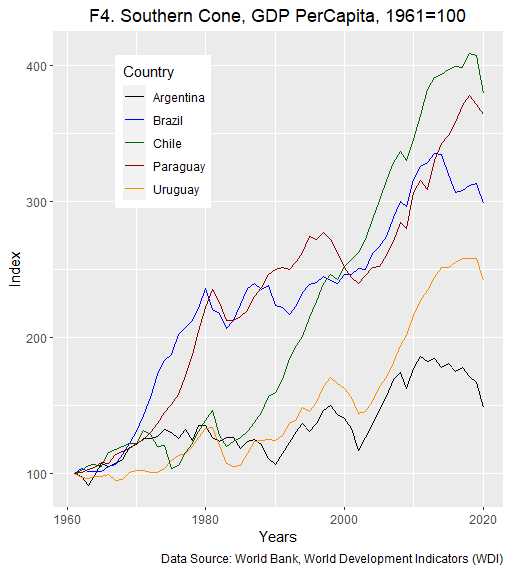
From the “Catch-up” post, we saw that the two highest growth countries in the 1960-2020 period were China and South Korea. Figures 5 and 6 below show how their growth trajectories compare. Their growth rates were comparable until the early 1990s, when South Korea’s growth rate slowed down and China continued its accelerated pace.
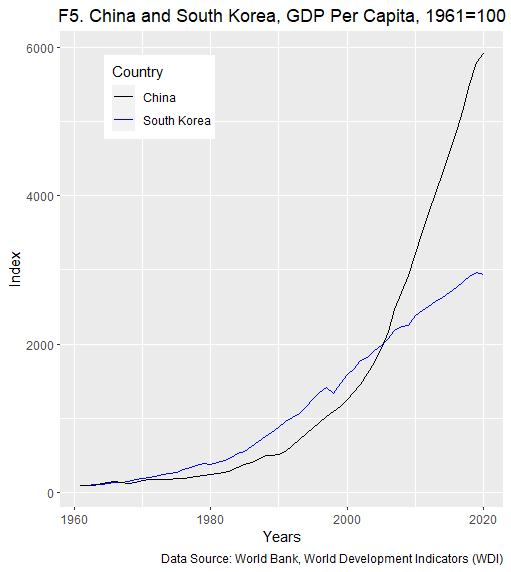
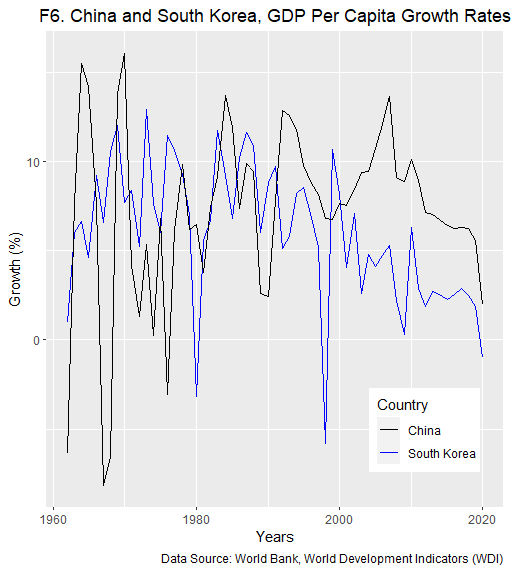
Two other interesting pairs of growth trajectories are the United States and the United Kingdom; and Bolivia and Guatemala. After the five aforementioned European countries, the next closest pair of growth trajectories are those of the United States and the United Kingdom (F7). All other countries with trajectories similar to others in 50 or more of the 59 years compared are rich countries (other European countries and Australia), the exception being the pair of trajectories for Guatemala and Bolivia (F8). Both these countries saw their GDP per capita fall in the first half of the 1980s. I will leave the reasons to explore in a potential future post.
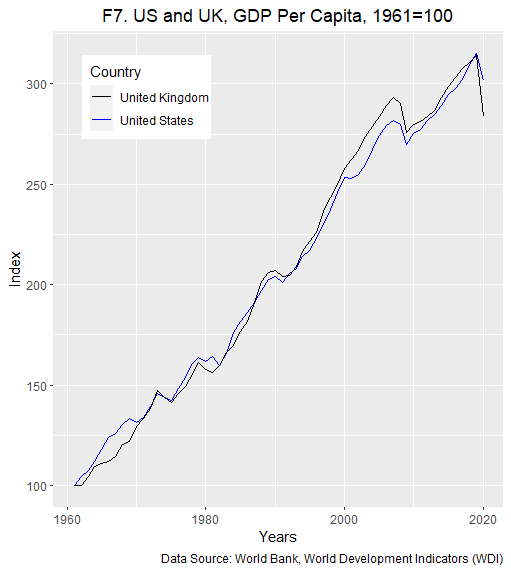
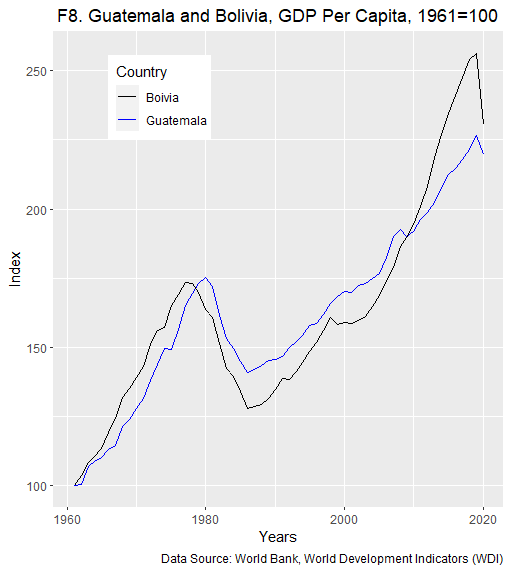
The exercise above suggests strong connections between the growth trajectories of rich countries, not as much for the rest of the world. It also proved to be a nice little contribution to my own R learning. I intend to further explore growth data in future posts.
References
World Bank: World Development Indicators. Available from USAID IDEA: https://idea.usaid.gov/. Accessed: January 14, 2023